Ensuring Authenticity: How Amazon Leverages AI to Combat Fake Reviews
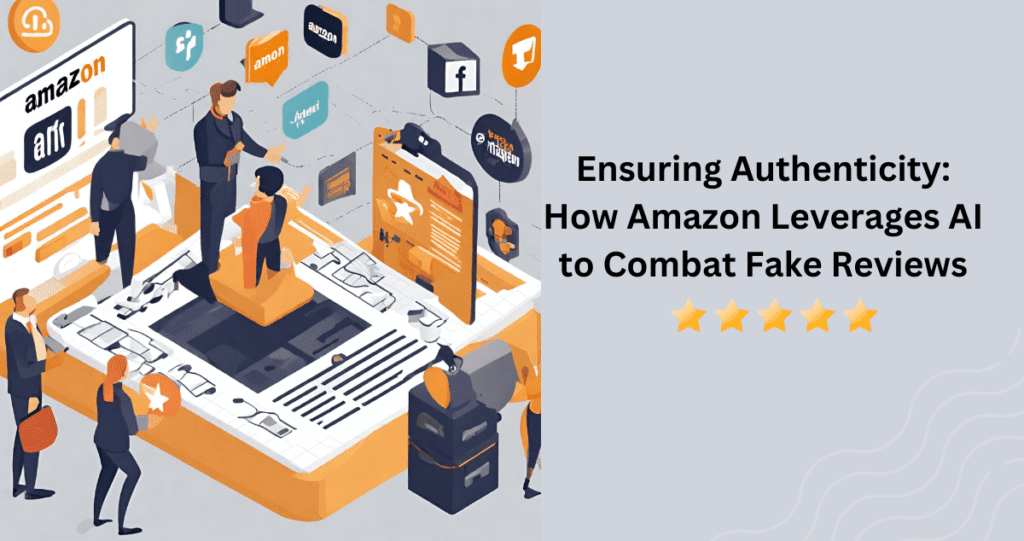
Introduction:
Customer reviews play a pivotal role in shaping purchasing decisions, and Amazon has been a frontrunner in harnessing the power of artificial intelligence (AI) to ensure the authenticity of reviews on its platform. Since its inception in 1995, Amazon has strived to make it effortless for customers to share honest feedback while concurrently fortifying its defenses against fraudulent activities that could compromise the trustworthiness of its review system.
Analyzing Reviews with AI:
Upon submission, every customer review undergoes a rigorous analysis through advanced AI algorithms before being published online. Amazon’s AI system scrutinizes the reviews for known indicators of potential fraud, allowing the majority of authentic reviews to be swiftly posted. However, in cases where suspicious activities are detected, Amazon employs a multi-faceted approach. If a review is unequivocally identified as fake, prompt action is taken to block or remove the review. Further measures may include revoking the reviewer’s permissions, blocking associated accounts, and even pursuing legal action against offenders. In 2022 alone, Amazon proactively thwarted over 200 million suspected fake reviews worldwide.
Proactive Measures and Expert Investigators:
Amazon invests significantly in proactively preventing fake reviews from reaching customers. Utilizing the latest advancements in AI, machine learning models analyze diverse proprietary data sets. This includes factors such as seller ad investments, customer reports of abuse, behavioral patterns, and review history. Amazon’s expert investigators, specially trained to identify abusive behavior, play a crucial role in cases where additional evidence is required. Their expertise aids in distinguishing between authentic reviews and those intended to mislead customers.
Advanced Technologies Deployed:
Amazon leverages a combination of machine learning models, natural language processing techniques, and deep graph neural networks to combat fake reviews comprehensively. The models analyze anomalies in data, such as rapid review accumulation due to advertising or competitive pricing. Additionally, they scrutinize linguistic patterns to identify potential fraud, including reviews incentivized with gift cards or free products. Deep graph neural networks are instrumental in understanding complex relationships and behavioral patterns, assisting in the identification and removal of groups involved in deceptive practices.
Addressing Misconceptions:
Josh Meek, Senior Data Science Manager on Amazon’s Fraud Abuse and Prevention team, emphasizes that differentiating between authentic and fake reviews is not always straightforward for external observers. Critics who challenge Amazon’s review detection methods often overlook the intricacies involved. Meek explains that the combination of advanced technology and proprietary data allows Amazon to identify fake reviews accurately by delving deeper into the underlying relationships between bad actors, surpassing surface-level indicators.
Conclusion:
Amazon’s commitment to maintaining the integrity of customer reviews is evident in its robust use of AI technologies. By employing cutting-edge algorithms, machine learning models, and expert investigators, Amazon remains at the forefront of the battle against fake reviews. This not only safeguards the trust of millions of customers relying on authentic reviews but also ensures a fair and reliable platform for honest sellers. As technology continues to advance, Amazon’s ongoing efforts to stay ahead in the fight against fraudulent activities serve as a testament to its dedication to providing a trustworthy shopping experience for its global customer base.
Recent Posts
- Mastering Amazon Product Photography: Key Considerations for Optimal Results
- Unlocking Success: The Importance of Amazon Listing Optimization for Increased Sales
- A Guide to Amazon Listing Optimization in 2024
- Capturing Commerce: The Impact of Product Photography on Ecommerce Growth
- A Complete Guide to E-commerce photography, Tips and Tricks